Problem
The Li-ion traction battery (LIB) is currently the most valuable component in an electric vehicle, accounting for 30-50% of costs. As an electrochemical system, LIBs suffer from degradation mechanisms, which is why their state of health deteriorates over time and use. At a certain state of health (e.g. at 80% remaining capacity), the automotive requirements can no longer be met, so the battery must be replaced. The time of replacement and the associated service life of LIB is a decisive factor in the economic and ecological profitability of electric vehicles. A precise determination of the aging condition and prediction of the remaining service life of LIB is therefore desirable for battery and vehicle manufacturers as well as for fleet and end customers. However, the prediction of LIB lifetime is a difficult task and currently poses a major problem. The currently used models are a) too inaccurate and b) too complex and expensive to create.
Goal
The bawaii project aims to achieve a breakthrough in the lifetime prediction of Li-ion battery packs for electric vehicles. The growing number of electric vehicles and the associated spread of Li-ion batteries allows the collection of large amounts of battery specific data during operation. The aim of the project is to use these data for a reliable and precise aging and life cycle prediction of Li-ion traction batteries along their life cycle. This will be achieved by developing a cloud-based hybrid AI software. Currently used empirical aging models do not map certain system and statistical scattering effects, which is why they are becoming increasingly inaccurate over vehicle usage. In contrast, the model accuracy of data-based models increases with increasing lifetime and data volume. Behind the idea of hybrid AI software lies the combination of the complementary advantages of both modeling domains. Besides the pure utilization of data, the artificial intelligence is to be extended with battery specific know-how from empirical ageing models. The potential uses of hybrid AI software for lifetime prediction are manifold, because it helps all stakeholders along the value chain such as OEMs, battery system manufacturers, fleet operators and lessors to save costs and time:
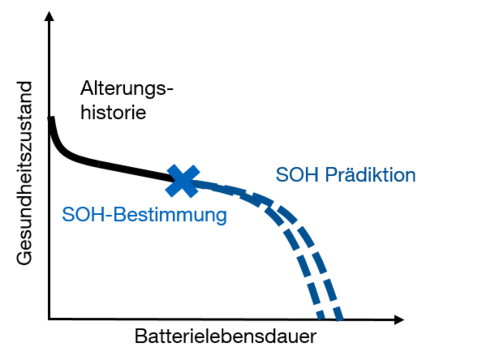
- Increase of the usable range
- Extending battery life
- Predictive maintenance
- Increased efficiency in the development process
Implementation
The consortium consists of the Chair of Automotive Engineering (FTM) of the TU Munich and the young Bavarian technology company TWAICE Technologies GmbH (spin-off of the TU Munich). The distribution of tasks is additionally illustrated by the diagram in Fig. 3.
Chair of Automotive Engineering: The FTM is responsible for the scientific support, consolidation and validation of the project. This includes the fundamental research of AI methods for the lifetime prediction of LIB and the extension of the AI by domain-specific know-how from empirical approaches. A consideration of individual physical aging mechanisms in AI models has to be investigated and a model definition has to be developed that meets the requirements.
TWAICE: TWAICE is responsible for data management, including adaptation of the complete technology chain for data acquisition, preprocessing, transmission and storage in a server backend. The main focus is on the appropriate provision of input parameters and the determination of real output parameters for the validation of forecasts. On the other hand, TWAICE is responsible for the implementation of the AI platform, continuous learning/adaptation/further development of the AI and testing of the newly developed method in real fleet tests.
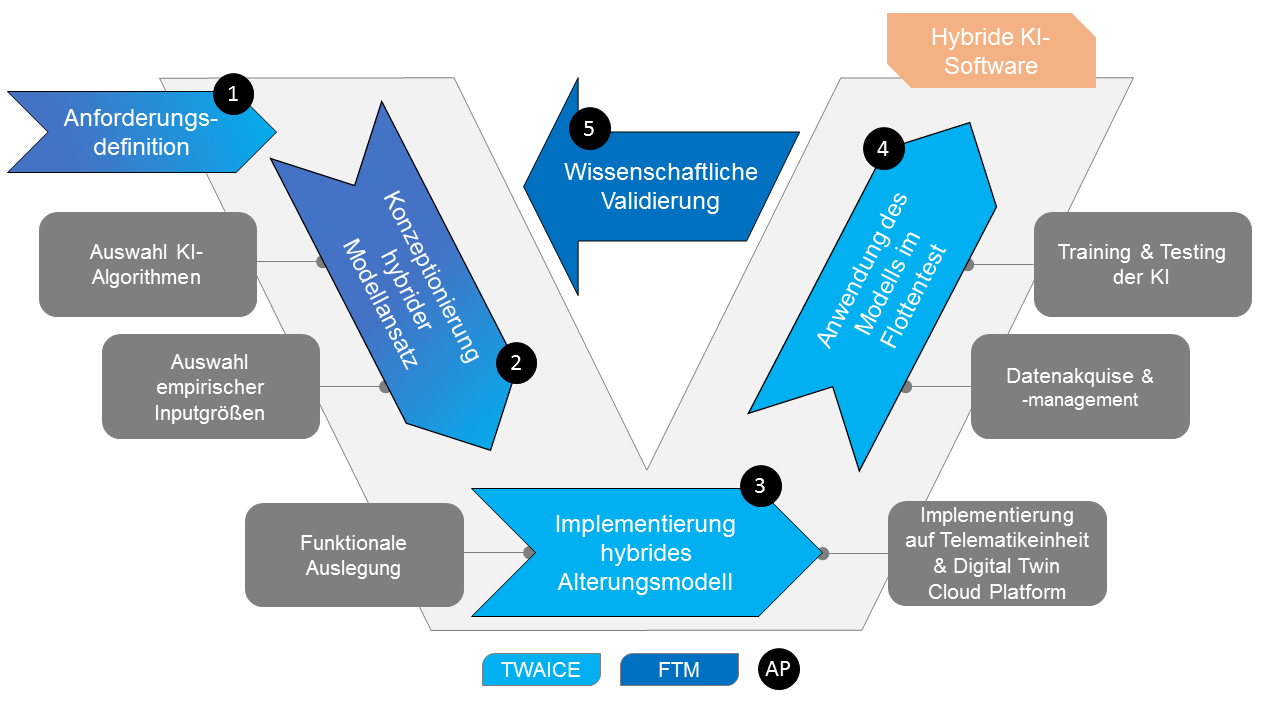
Based on the V-model for software development, the overall project is divided into the main work packages Requirements Definition (WP 1), Conceptualization of Hybrid Model Approach (WP 2), Implementation of Hybrid Aging Model (WP 3), Application of the model in fleet testing (WP 4) and Scientific Validation (WP 5) (see Fig. 5). The hybrid AI software is to be developed as a technical product. The structuring of the work packages on the basis of the V-model results in important iteration loops between the individual development phases, which should ensure the best possible implementation of the research project from a technical point of view.