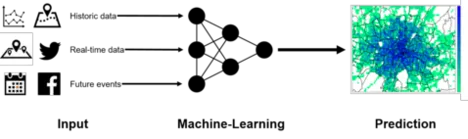
Problem
Future technologies such as electromobility, networking and autonomous driving are leading the classical mobility concepts into the territory of “Mobility as a Service” (MaaS). In the future, this will require the traditional mobility service providers to reconsider their strategies.
This advancement is particularly visible through the example of the taxi industry:
In the past, the taxi industry in Germany enjoyed a certain monopoly over the individual transport of people. Recently, however, an increasing number of competitors have been entering the market to challenge the conventional taxi operators and general operation centers.
These developments force the fleet operators and the operating centers to increase their efficiency in order to remain competitive in the long term. A high potential for improvement here can be found in the reduction of waiting times of taxi drivers. Currently, in average a taxi is in use around only 40% of the day. It is expected that with an accurate passenger traffic forecasting in combination with a suitable fleet strategy, this ratio can be dramatically increased.
Goal
The focus of this project lies on the exploration of spatial and temporal passenger volume for on-demand mobility offers. Using the example of Munich’s taxi business, a model for the prediction of the passenger volume is to be developed. In contrast to the previous approaches, with the use of machine learning and extended input parameters the accuracy of the predictions should be both spatially and temporally increased. In a second step, the layout of a convenient fleet strategy will take place.
Research Questions
- Which spatial / temporal factors influence the demand for on-demand mobility services?
- How can a highly spatial and temporal accuracy in the prediction be achieved by merging multiple data sources?
- What will be the optimal fleet strategy if the demand can be predicted with a sufficient accuracy?
Approach
Data Acquisition & Analysis
For the analysis of the passenger traffic, the Institute of Automotive Technology has the data set of a Munich taxi center. The data currently covers trips with a total length of 55 million kilometers and around 11 million single trips. The data set is then updated in 10-minute intervals with current data from the fleet management of the taxi center.
Modelling
For the modeling of the demand behavior machine learning will be used. The future versions of the model should be able to predict the passenger volume by taking into account the historical and current data, as well as the known future events.
Verification and Plausibility
The verification of the model will be done using historical data. For this purpose, the data set will be separated into training and validation sets.
Finding the Optimal Fleet Strategy
Based on the results of the prediction model, a final analysis should reveal how an adapted fleet strategy can improve the performance of the overall system while maintaining the same service quality.