Estimation and Prediction of the tire-road friction potential of an autonomous racecar
Project Completed - Contact: ftm(at)ftm.mw.tum.de
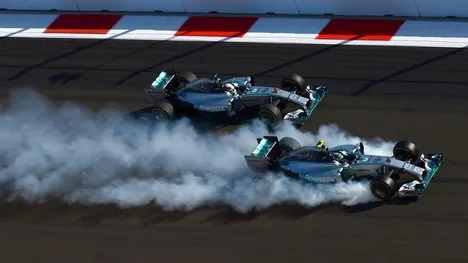
Motivation
The coefficient of friction μ prevailing during the interaction between a vehicle’s tires and the road surface has a major influence on driving behavior and driving safety. The conditions at the contact point determine the maximum transferable acceleration, braking and lateral forces of the vehicle. The so-called friction potential depends on numerous influencing variables and cannot be measured directly at the point of contact, but can only be estimated.
Due to the high relevance for driving safety, precise knowledge of the maximum transferrable forces and thus knowledge of the friction potential plays an important role. Especially in the context of the increasing automation of driving functions, the vehicle itself must be able to estimate the friction potential as accurate as possible. This is particularly essential in emergency situations, where the maximum coefficient of friction must be exploited in order to avoid any damage or injuries.
In motorsport, there is another important aspect in addition to the mentioned safety aspect. The human racing driver constantly strives to utilize the full tire force potential in order to achieve minimal lap time. The friction potential determines the maximum possible acceleration/deceleration in longitudinal direction and the maximum possible lateral acceleration of the racing vehicle. The influence of the coefficient of friction on the vehicle’s driving dynamics and the achievable lap time is significant. The exploitation of the friction potential should therefore be maximized in every situation. However, overdriving the limit must be prevented at any time, but time safety margins must also be kept as small as possible to avoid performance loss due to conservative driving.
Objective
The goal of this research project is the development of a real-time algorithm, which is able to estimate the friction potential of the tire-road contact and - especially with regard to racing - to predict it for different planning horizons. The friction potential should be determined with high local resolution for the entire racetrack in order to take factors into account, which only locally influence the coefficient of friction (e.g. drying track, varying road surface). The resulting high-resolution map can significantly increase the performance of the racecar. When transferring the algorithm to road traffic, precise knowledge of the friction potential helps to act appropriately in safety-critical situations and to achieve a safe driving condition.
Realization
First, a comprehensive literature search is carried out in order to identify the already established methods for estimating and predicting the friction potential in the given field of application. In addition, machine learning approaches will be researched to determine whether they are suitable for estimating and predicting the friction potential in the given field of application and whether they can be applied to it. Subsequently, methods from both categories (conventional and machine learning) will be implemented, compared and further developed. In addition to the application in a simulation environment, the most promising methods will be applied to a real racing car and their functionality will be evaluated based on performance parameters from racing.