OptiPro - Optimized cell finalization through innovative plant technology and parameter-supported evaluation of process and quality parameters in the virtual production system
Contact: Manuel Ank, M.Sc.
Problem
The production of lithium-ion battery cells is completed in the process steps of cell finalization. Up to now, cell finishing in battery cell production has been characterized by limitations in the achievable throughput and insufficiently known influence on the cell quality parameters. Among other things, this is reflected in long process times and high production costs.
Goal
The aim of the research project is to realize optimized cell finalization through innovative plant technology and parameter-supported evaluation of the process and quality parameters in the virtual production system. For this purpose, the entire process chain of cell finishing is to be digitized using state-of-the-art technology. This means that innovative measurement technology will be used to enable a parameter-based evaluation of the process and quality parameters of the respective process step. The data will be mapped in a virtual production system and analyzed and evaluated using artificial intelligence (AI). All plant technologies used within the cell finishing process are to communicate between the real and virtual production systems in such a way that a permanent feedback loop is created.
The large energy and resource requirements of some sub-steps (including filling, forming, test storage and end-of-line test) motivate to minimize process times. The material demand represents a significant cost driver, subsequently a targeted reduction of scrap is driven by process optimization. Through the exchange of information between real and virtual plants, this effort to reduce process times and increase quality is to be achieved.
The research project thus has great potential to strengthen the attractiveness of competitive battery production in Germany by influencing cell finishing in a holistic manner.
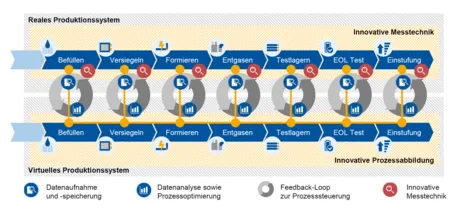
Implementation
In the research project, the Institute of Automotive Technology makes a decisive contribution to the application-specific definition of the target image and focuses the research activities on the implementation and optimization of end-of-line (EOL) testing and downstream validation of the methods and models through extensive life tests. The aim is to achieve significant process time reductions in EOL testing through the cross-process use of inline-capable measurement technology, new measurement methods and a model-based link, and thus
- to achieve shorter throughput times of individual process steps,
- identify and eliminate defective cells at an early stage, and
- to achieve more precise key figures for cell quality
in order to consequently minimize the use of resources and thus reduce production costs. The metrological link within a virtual production system also enables optimized control and regulation of the process steps in order to increase the quality of production in the long term through real-time capable cross-process control interventions.
Project classification
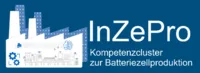
The project is part of the BMBF competence cluster 'Intelligente Batteriezellproduktion' (InZePro). The focus is on increasing the productivity of cell production and making it more flexible. This is to be achieved through holistic optimization of the production system using Industry 4.0 solutions, focusing on innovative agile plant technology, digitization, artificial intelligence (AI) in production and virtual production systems. More info here.
Project partners
In the battery competence cluster "Intelligente Batteriezellproduktion" (InZePro), it was decided to fund the research project by the Federal Ministry of Education and Research (BMBF). The Institute Production Engineering of E-Mobility Components at the RWTH Aachen University (PEM - RWTH), the Institute for Power Electronics and Electrical Drives at the RWTH Aachen University (ISEA - RWTH), the Institute for Information Management in Mechanical Engineering at the RWTH Aachen University (IMA - RWTH), the Institute for Machine Tools and Industrial Management at the Technical University of Munich (iwb - TUM) and the Institute of Automotive Technology at the Technical University of Munich (FTM - TUM) are conducting joint research.
This research project is funded by the BMBF (grant number 03XP0364B) and cared by Project Management Jülich. The responsibility for the content of this publication lies with the author.


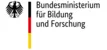
