Calibrating Transport Models
As George E. P. Box once said “All models are wrong, but some are useful”. Transport models are used by the government to design new infrastructures and answer key questions such as:
“What will happen if we build a new road?”
“What if we ban cars in city centres?”
The ‘usefulness’ of a transport model depends on how good the model is in answering these questions. Model Calibration uses data to validate and correct a transport model and makes informed decision-making possible. The Chair of Transportation Systems Engineering (TSE) at the Technical University of Munich is a worldwide leading research group on this topic, with contributions encompassing real-time applications, large scale applications, innovative problem formulations, and using Artificial Intelligence.
The Problem at a Glance
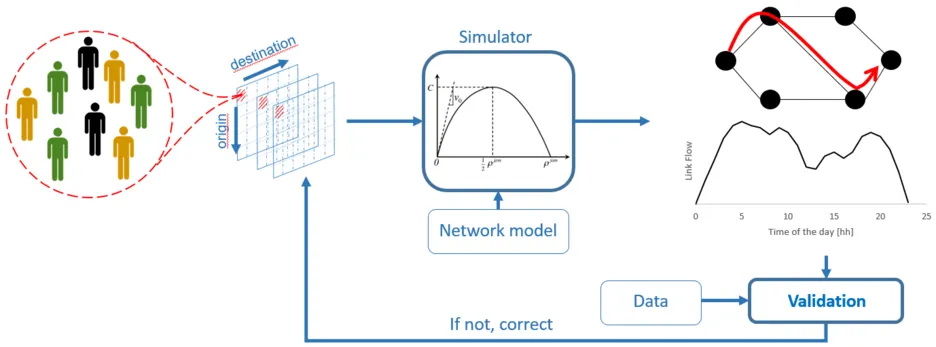
The main scientific challenge arises from the fact that transport systems are extremely complex and data can only partially help into understanding where the problems are. This is particularly true for the demand. Conventional monitoring systems, such as loop detectors, measure the effect of the demand on the network rather than the demand itself. More advanced techniques, such as GPS tracking and plate number recognition, provide better insights into the number of trips performed in a transport network. Therefore, state of the art solutions perform fair rather than good. The situation gets significantly worse for large networks, such as the regional network of Munich.
Applications
Dimensionality reductions techniques: Researchers at TSE are now working with dimensionality reduction techniques and concepts from artificial intelligence to calibrate this network, one of the largest ever calibrated until today. Our current models can handle such a network, achieving errors as low as 10%, but more work is still needed to (i) reduce computational times and (ii) reproduce complex traffic dynamics. See [1-4] for more information.
Activity based demand estimation: Incorporating information about daily activity scheduling and duration is of paramount importance to derive dynamic OD flows. To achieve this goal, researchers at TUM developed new frameworks that take into account the underlying daily and weekly structure of the demand to obtain more robust estimations. By introducing a parametric representation of the demand, strong correlations between variables can be exploited to better understand complex dynamics such as the correlation between commuting and not commuting demand. See [2,5] for more information.
Data driven model calibration: At TSE, we also believe that there is an urgent need for bridging the gap between machine-learning and traditional demand modelling. Artificial Intelligence can in fact help to better understand the data. For instance, contextual data (social media, weather data) can be successfully used to identify mobility patterns from large data-sets of data. This has been shown for the case of New York city, where more than 4.000.000 trips were analysed. See [3] for more information.
Work with Us
Model Calibration is a collaboration between our research groups "Transport Data Analytics" and "Transport Optimization" at TSE. You can refer to (in alphabetical order) Dr. Guido Cantelmo, Mahajan Vishal, and Qurashi Moeid for more details on our most recent works. If you are interested in machine learning, data mining, traffic simulations, please contact us for research topics for bachelor, masters thesis, etc.
Key Publications
-
Qurashi M., Lu Q. L., Cantelmo G., and Antoniou A. “Dynamic demand estimation on large scale networks using Principal Component Analysis: The case of non-existent or irrelevant historical estimates,” Transp. Res. Part C Emerging Tech., March. 2022. https://doi.org/10.1016/j.trc.2021.103504
-
Cantelmo, G., Qurashi, M., Prakash, A. A., Antoniou, C., and Viti, F. “Incorporating trip chaining within online demand estimation,” Transp. Res. Part B Methodical., Jun. 2020. https://doi.org/10.1016/j.trb.2019.05.010
-
Cantelmo, G., Kucharski R., and Antoniou C. “A Low Dimensional Model for Bike Sharing Demand Forecasting That Explicitly Accounts for Weather Data.” Transportation Research Record 2020: 0361198120932160
-
Qurashi, Moeid, et al. "PC–SPSA: Employing dimensionality reduction to limit SPSA search noise in DTA model calibration." IEEE Transactions on Intelligent Transportation Systems 21.4 (2019): 1635-1645.
-
Cantelmo, Guido, Francesco Viti, Ernesto Cipriani, and Marialisa Nigro. "A utility-based dynamic demand estimation model that explicitly accounts for activity scheduling and duration." Transportation Research Part A: Policy and Practice 114 (2018): 303-320.
15.02.2021 by Guido Cantelmo, Moeid Qurashi, and Vishal Mahajan