Laura Gualda
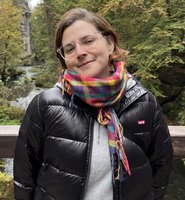
Laura Gualda is a Research Associate and Ph.D. candidate at the Chair of Transport Systems Engineering at the Technical University of Munich (TUM) under the supervision of Prof. Dr. Constantinos Antoniou since February 2024. Her current research focuses on uncertainty quantification in machine learning frameworks, transfer learning methods for traffic flow estimation and transport-related air pollution.
Prior to her current position as Research Associate, Laura worked for five years in data engineering and analytics in the private sector in Berlin. She holds an M.Sc. in Mathematical Data Modelling from the School of Applied Mathematics of Fundação Getulio Vargas (Rio de Janeiro, Brazil) and a B.Sc. in Economics from the Brazilian School of Economics and Finance of the same institution.
Education and Training
2024 - present | Ph.D. Candidate Chair of Transportation Systems Engineering Technical University of Munich (TUM), Munich, Germany |
2016 - 2018 | M.Sc. Mathematical Data Modelling Coursework: Data Mining; Multivariate Statistics; Machine Learning; Monte Carlo Simulation Methods Thesis: Learning about corruption: a statistical framework for working with audit reports |
2012 - 2015 | B.Sc. Economics Focus on Econometrics and Causal Inference |
Work Experience
2024 - present | Research Associate at the Chair of Transportation Systems Engineering, TUM, Munich Working on the MI-TRAP project |
2022 - 2024 | Data Engineer at DeepL SE, Berlin |
2020 - 2022 | Data Engineer at Delivery Hero SE, Berlin |
2018 - 2020 | Fraud Data Analyst at Fraugster GmbH, Berlin |
2017 - 2018 | Machine Learning Graduate Intern at IBM Research, Rio de Janeiro, Brazil |
2012 - 2017 | Research and Teaching Assistant at Fundação Getulio Vargas, Rio de Janeiro, Brazil |
Publications
Reinaldo Mozart Da Gama e Silva, Laura Gualda, Lucas F. Lima, Emilio A. Vital Brazil, Renato F. G. Cerqueira, Rogério A. Paula and Ulisses T. Mello (2018). Sensitivity Analysis in a Machine Learning Methodology for Reservoir Analogues. Rio Oil & Gas Expo and Conference Proceedings, p. 24-27, 2018.